Conceptual overview
The task of industrial processing is to create products with specific functional, textural and sensory properties from basic materials. Given the complexity of this endeavor, systematic investigations on multiple length and time scales are required to design and operate the next generation of engineering processes (Fig. 1).
Since production very often takes place in enclosed machines, experimental data collection is often limited. On the other hand, computational fluid dynamics (CFD) simulations can provide valuable additional information throughout the process domain, especially when advanced microstructure-based models are used and iteratively developed through frequent back-testing against experimental data. Machine learning using real-time mechanical data with miniaturized, customized machining setups, advanced sensor technology used in pilot-scale processing, and 3D data from CFD process simulations is an effective tool for process design, optimization, and control (Fig. 2)
The focus of our research is on the industrial challenges of the 21st century. Today's manufacturers are faced with a highly competitive global market, the needs of a rapidly growing population and limited environmental resources. To succeed in such a market, processes must be optimized and novel products must be manufactured to meet the high demands of consumers. Such products tend to be more complex and sometimes have smart properties that require dynamic adaptation of certain material properties in response to environmental changes or the presence of enzymes or chemicals. In the following, we present in more detail how we address these challenges using a multiscale approach to process systems engineering.
- Physics-based CFD simulations of engineering processes. Using physics-based arguments, we develop microstructure-based CFD models for large-scale process simulations. Complex changes in material properties associated with temperature, chemical or enzymatic reactions during processing will be considered as well as the implementation of intelligent product functions. These developments form the basis for future high-performance computational applications related to the design and optimization of complex unit operations, 3D tissue printing, soft gel encapsulation, multilayercoatings with functional particles, wet steam cleaning, among others.
- In-situ real-time multi-scale material characterization. It is crucial for process engineers to know how the internal structure and mechanical properties of a material change dynamically in response to an imposed deformation or an external trigger such as the addition of an enzyme or a crosslinking agent.
Using tailored mechanical experimental setups in combination with state-of-the-art optical (SAXS, SANS, μ-CT, FBRM) and chemical analysis techniques (Raman spectroscopy), we monitor material changes down to molecular structures in situ and in real time under well-defined deformation conditions (shear/strain/compression) and in miniaturized devices during processing operations. Examples of such processes include 3D printing, spray drying, mixing, and filtration. To analyze processes in standard pilot plants, state-of-the-art sensors (e.g. image analysis, particle size and count, chemical composition) are placed at specific points in the process equipment to collect data.
The knowledge gained from these studies can be useful for the development of advanced microstructure-based CFD models, the fabrication of materials with smart functions, and the design and optimization of process flows.
- Data-driven process systems engineering. With the rapid increase in computing power of hardware in recent years, machine learning (ML) has become increasingly popular for technological applications. ML algorithms rely heavily on mathematical concepts of data analysis and are particularly useful in complex situations where other approaches fail.
When combined with advanced machine learning techniques, CFD can become even more powerful. For example, my CFD simulations provide valuable insight into the internal structural and macroscopic properties at all points in the flow field. To determine whether or not the physical hypothesis underlying my CFD models is correct, and if so, how to further improve its predictive power, we perform real-time multiscale material characterizations in situ, similar to those described above, and analyze the results using ML tools such as MATLAB, TensorFlow, and Scikit-learn. In addition, ML allows us to use multiple datasets and a larger number of different deformation protocols for CFD parameter estimation, which provides a more realistic representation of average material behavior.
In addition, we apply ML to the collected in situ mechanical data, sensor data, and CFD data to identify and control for the various factors (e.g., processing equipment design, processing conditions, etc.) that affect the final product material properties and process performance.
Contact us
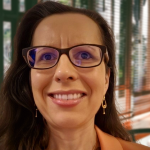
Natalie Germann
Univ.-Prof. Dr.Head